| 2025 VOLUME 8, ISSUE 2, MARCH - APRIL
Remaining papers are under processing.....
S.No. |
March - April |
Page No. |
Downloads |
1. |
Advancing Aneurysm Neck Healing: Evidence from Drug-Eluting Stent Technologies
Ruiliang Wang 1, Jun Wen 2
DOI: https://doi.org/10.56293/IJASR.2025.6401
ABSTRACT: Subarachnoid haemorrhage resulting from intracranial aneurysms (IAs) represents the third most
prevalent acute cerebrovascular disease with high mortality and disability rates, following ischaemic stroke and
hypertensive intracerebral haemorrhage. Cerebral aneurysms pose a significant threat to public health in China,
imposing substantial economic burdens on families and society. Current treatment modalities predominantly include
surgical clipping and endovascular intervention, with the latter emerging as the primary therapeutic approach.
Studies indicate that standalone coil embolisation is associated with a slightly higher postoperative recurrence rate
compared to stent-assisted coiling, largely due to incomplete endothelial coverage at the aneurysm neck. To enhance
endothelialisation at the aneurysm neck and mitigate risks of rupture and recanalisation, recent research has focused
on applying advanced coating materials to bare metal stents, aiming to promote neointimal formation at the
diseased arterial segment. This article provides a retrospective analysis of the efficacy of various stent coating
materials in facilitating postoperative endothelialisation at the aneurysm neck.
Keyword: Drug-eluting stents, Endothelialisation, Intracranial aneurysm recurrence
Download full manuscript.......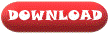 |
01-14 |
 |
2. |
Adapting to the new normal: the dilemma of wearing face mask
1 Neha Kumari, 2 Prof. Rajni Dhingra
DOI: https://doi.org/10.56293/IJASR.2025.6402
ABSTRACT: The sudden emergence of COVID-19 in 2020 revealed a new aspect of face masks. From a medical
supply to an essential preventive measure, face mask has marked their era. Face mask usage patterns and practices
are determined by the mask perception of an individual. Various research studies have highlighted the influence of
age group, gender, and location on these perceptions. Mask resistance is prevalent among the general public even
after the tragic waves of COVID-19. It becomes important to understand the barriers and motivating factors of
face mask usage during the pandemic as it provides reliable justification for mask resistance. The present paper is an
attempt to highlight various factors that affect the usage of the facemask to deal with the pandemic during the
pandemic and in the post-pandemic (so-called ‘New Normal’ era). Even as the pandemic moves into an endemic
phase, it is still important to wear masks, particularly in environments where vulnerable populations like the elderly
and those with weakened immune systems are present. Face mask, the new skill of living, requires mandatory
adoption to work efficiently in the post-pandemic era or new normal. New normal signifies regular adoption of all
necessary preventive measures to step forward in the pandemic times. The present research has implications for the
adoption of preventive strategies during the new normal phase.
Keyword: COVID-19, face mask usage, barriers, motivating factors, new normal
Download full manuscript.......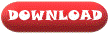 |
15-21 |
 |
3. |
ZEBRAFISH RESEARCH IN INDIA- A BIBLIOMETRIC ANALYSIS
Kokilamani A. L. 1*, Sreedhar H. K. 1, Sindhura Y.M. 1, Usma Khanum 1, Mythra N. 1, and Shashidhara M. 1
DOI: https://doi.org/10.56293/IJASR.2025.6403
ABSTRACT: Zebrafish (Danio rerio) is a prominent model organism in biological research in recent times. To know the
current trend of research topics, scientists, Universities/institutions, funding organizations in the Zebrafish research
has become our priority to take up this study. The study included the collection of articles on Zebrafish research
published between 2000 to 2024 (July) particularly from Indian Authors through online databases-PubMed,
Scispace, Web of Science, further transformed the collected bibliometric data into quantitative data and analysed it
by using software tool RStudio-Biblioshiny-Bibliomatrix. From the study it has revealed that a total of 1,807
documents published from 2000 to 2024 in 672 journals, books etc., at 24.81% of annual growth rate with 18.57
average citations per document involving 8,109 authors from various institutions/University across the nation. In
India UGC, and DBT are the leading funding agencies for zebra fish research. Our study provides a comprehensive
and current trend on Zebra fish research in India and is baseline information to young researchers to conduct their
experiments in new trend areas of zebrafish science and further Universities/institutions and funding agencies make
informed decisions.
Keyword: Zebrafish, Bibliometric analysis, model organism, Zebrafish research in India
Download full manuscript.......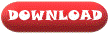 |
22-32 |
 |
4. |
EXPLORING THE INTERSECTIONALITY OF GENDER AND AGRICULTURE USING CREATIVE ART: A HUMAN RIGHTS-BASED APPROACH FOR THE EMPOWERMENT
OF WOMEN IN RURAL NIGERIA.
Dr.Grace Chizoma, Onyebuchi-Igbokwe, Dr. Rachel Nkeonyere, Nwakasi, Dr. Chizoba Anderson Egornu, Dr. Jovita Charles Ogu, Dr. Bertha Oluchi Uzowuihe, Dr Peace Chimarauche Amanze, Prof. Edem Peters
DOI: https://doi.org/10.56293/IJASR.2025.6404
ABSTRACT: Nigerian rural women face particular opportunities and problems due to the interaction of gender and
agriculture. Through a human rights-based perspective, this study investigates how creative art might be used as a
tool to combat gender inequality and empower women. A thorough conceptual model, a variety of methodological
tools, and theoretical foundations are all included in the enlarged framework. The study examines how well artistic
interventions work to raise awareness, improve skills, and advance gender parity using data gathered from Nigerian
rural communities. The results of the statistical analysis, which was carried out using SPSS (Statistical Package for
the Social Sciences), show that women's involvement in agricultural decision-making and revenue creation has
significantly improved. Rural women's voices are amplified through creative art, which includes visual arts,
performance, and storytelling, which depicts their goals, challenges, and lived experiences. This strategy encourages
discussion and group action for change in addition to increasing awareness of gender inequality by including the
community in participatory art projects. This study looks at how artistic expression can inspire grassroots initiatives
that support women's rights and empowerment, challenge gender conventions, and overcome cultural and
socioeconomic boundaries. The report also identifies important factors that contribute to empowerment, providing
practical advice for putting policies into practice.
Keyword: Intersectionality, Gender, Agriculture, Creative Art, Human Rights-based Approach, Empowerment,
Women, Rural Nigeria
Download full manuscript.......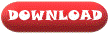 |
33-41 |
 |
5. |
ROLE OF AGRICULTURAL EXTENSION SERVICE IN THE ADOPTION OF
INTEGRATED PEST MANAGEMENT PRACTICES AMONG COCOA FARMERS IN
ONDO STATE, NIGERIA.
Akinwalere. B.O
DOI: https://doi.org/10.56293/IJASR.2025.6405
ABSTRACT: The study was on the adoption of Integrated Pest Management (IPM) Practices among Cocoa Farmers in
Owo Local Government Area, Ondo State, Nigeria. Specifically, the study aimed at ascertain the socio-economic
characteristics of the respondents in the study area, assess the level of awareness of integrated pest management
practices available to the respondents and evaluate the effectiveness of agricultural extension services in information
dissemination of Integrated Pest Management practices. Primary data were collected by administering a wellstructured questionnaire consisting of closed and open-ended questions. Multistage sampling was employed in the
study for the selection of 100 cocoa farmers in the study area. Data were analyzed using descriptive and inferential
statistics. The results showed that majority (84%) of the farmers were male, the mean age of the respondents was 47
years with an average household size of 7 members. The major educational qualification of the respondents was
secondary school (47%). Ninety-four percent of the respondents practiced Integrated Pest Management (IPM) and
89% have access to extension services also affirmed that IPM techniques are part of the services rendered by the
extension workers, out of which 78% implemented one of more of the techniques recommended by the extension
workers in the study area. The most significant impact of extension services was felt in the area of improved
collaboration and networking (𝑥̅=2.03.) and increase in the utilization of biological and traditional methods of pests
control (𝑥̅=1.96.) The study recommends that government and agricultural agencies should establish comprehensive
training programs focusing on simplifying IPM practices and providing hands-on training. Also, provision of
affordable credit facilities and subsidies to cocoa farmers to alleviate the high cost of implementing IPM practices.
Keyword: Extension Services, Adoption, Integrated Pest Management, Cocoa farmers
Download full manuscript.......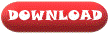 |
42-50 |
 |
6. |
USING LOW COAST MATERIAL (SEA GRASSES) AS ADSORBENT FOR REMOVAL ERIOCHROM BLACK T DYE FROM AQUEOUS SOLTIONS
1 HAMAD.M.ADRESS.HASAN, 2 ALIAA.M.M.KHALIFA, 1 HANAN .F.EMRAYED and
1 KAREMA.M.ABDEL-GANY 1
DOI: https://doi.org/10.56293/IJASR.2025.6406
ABSTRACT: Eriochrom T Black is one of most indicators used in different applications, especially for chemical
analysis it is a dye which mainly causes many problems from environmental media as a water aqueous solution. The
adsorbents of activated carbon used in this study were prepared from seagrasses. The kinetic behaviour of the
process was investigated. In order to study the adsorption efficiency, many parameters, such as contact time and the
adsorbent ratio, were used. Langmuir and Frundlish adsorption isotherm models were tested for the adsorption
process. The linear regression coefficient R2 was used to elucidate the best-fitting isotherm models. Adsorption
kinetics fitted well with the first-order kinetic model. The obtained results indicated succufilly adsorpition of the
studied dye using sea grasses activated carbon.
Keyword: Removal, Eriochrom.T, Sea grasses, Aquouse solution
Download full manuscript.......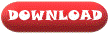 |
51-60 |
 |
7. |
DESIGNING AN AFFORDABLE AND ECO-FRIENDLY TIE-DYE SYSTEM FOR SMALLSCALE ENTREPRENEURS IN NIGERIA
Dr. Grace Chizoma Onyebuchi-Igbokwe., Prof. Eden Peters, Dr. Chizoba Anderson Egornu, Dr. Felicia Chinyere Okwulehie, Dr. Bertha Oluchi Uzowuihe, Nnamdi Chibuzo Adibe
DOI: https://doi.org/10.56293/IJASR.2025.6407
ABSTRACT: This study investigates the creation of an eco-friendly and reasonably priced tie-dye system specifically for
Nigerian small business owners. The study looks into economical material sourcing, ecologically friendly
procedures, and sustainable dying methods. Because of the use of synthetic dyes and high water use, the textile
sector is said to be a major contributor to environmental degradation. In order to promote sustainability and lower
the health hazards linked with harmful chemicals, it is imperative that eco-friendly alternatives be used. Both
qualitative and quantitative research approaches are used in this study to evaluate the suggested tie-dye techniques'
environmental impact and economic viability. The report also highlights how crucial consumer education is to
raising demand for eco-friendly textiles. While ANOVA identifies substantial differences in the environmental
impact between conventional and eco-friendly processes, SPSS data analysis indicates important cost reductions,
reduced environmental footprints, and enhanced market potential for sustainable textile products. The results
highlight the necessity of a comprehensive strategy that includes government regulations, educational initiatives, and
financial rewards to encourage the use of environmentally friendly dyeing techniques. This strategy also offers
insightful information to researchers, entrepreneurs, and policymakers about how to support sustainable textile
production and guarantee the financial stability of Nigerian small-scale craftspeople. According to the study's
findings, small business owners can successfully adopt sustainable tie-dye techniques, increasing their output and
promoting a greener economy, provided they receive the necessary assistance and education.
Keyword: Designing, Affordable, Eco-friendly Tie-Dye System. Small –scale Entrepreneurs, Nigeria.
Download full manuscript.......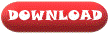 |
61-69 |
 |
8. |
A Comparative Evaluation of Optimal Geometric Camera Modelling Options for Airborne and Spaceborne Linear Array Cameras
Azubuike G. Nwosu, Raphael Ehigiator-Irughe, Jacob O. Ehiorobo
DOI: https://doi.org/10.56293/IJASR.2025.6408
ABSTRACT: Multiple variations of the collinearity model have been implemented for spaceborne SPOT and Airborne
ASAS imagery. The SPOT model showed better computational stability and achieved sub-pixel misclosures, in
some cases at checkpoints. The linear-phi parameter, excluded from some earlier SPOT models, indicated
significance and achieved improved performance. A model for airborne ASAS with 15 parameters achieved subpixel accuracy at GCPs only. A model utilising higher order polynomials as additional parameters in the airborne
model works well but does not render improved performance. INS measurements at 1Hz showed poor results,
proving to be inadequate for the imaging frame rate of 40 frames per second. With increasing GCP coverage,
misclosures at GCPs (internal accuracy) approach those at ties points (external accuracy).
Keyword: SPOT, ASAS, Camera Model, Linear Array
Download full manuscript.......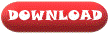 |
70-87 |
 |
|
More Links
For Authors
For Reviewer
|